Key Takeaways
- The Impact of Declining AI Costs on Investment Decisions
As AI training and inference costs continue to drop, the industry must reassess spending and capital allocation. DeepSeek’s advancements underscore this trend, challenging traditional assumptions about AI development and deployment cost structure. - Gains in Software and Hardware Efficiency Are Reshaping AI Economics
As larger compute clusters, enhanced power management, and networking improvements advance AI capabilities, optimizing costs has become equally important. Cost efficiencies, driven by necessity in regions with hardware constraints, are redefining the balance between absolute compute power and AI model performance. - Better Economics are Driving The Shift from Infrastructure to Applications
AI’s evolution is moving beyond infrastructure investments toward application-layer growth. Enterprise AI adoption, the rise of AI agents, and increased consumer utility are driving new use cases, influencing how capital is deployed in the AI ecosystem. - Democratization and Edge AI as Key Growth Drivers
Lower inference costs are accelerating the democratization of AI, making advanced models more accessible to developers and enterprises. Meanwhile, edge computing is poised for long-term expansion, with increased demand to support AI workloads on local devices.
DeepSeek – a China-based AI startup operating with limited resources -delivered the first major jolt to investors in the AI trade, triggering a sharp selloff that erased hundreds of billions from Nvidia’s market value. While the initial panic has subsided as tech companies provide guidance for the year, the broader implications are only beginning to take shape. DeepSeek’s open-source approach and claims of reduced computational costs have sparked debates in technical communities and investor circles about the future of AI development and deployment. We believe we are years away from considering the next AI winter and remain in the early stages of the AI cycle (see Appendix A). However, DeepSeek has challenged the dominance of Western AI leaders, drawing attention to China’s emerging AI contenders and raising questions about the trajectory of AI spending.
DeepSeek: Disruptor or Catalyst?
In January, DeepSeek released its two newest LLMs, V3 and R1. Building from prior models and using novel techniques, DeepSeek has achieved performance levels comparable to frontier models like OpenAI’s GPT-4 and o1, with an estimated pre-training cost of only $5.6 million1. This sharply contrasts with the increasing amounts spent on training the leading models in the U.S.2. Algorithmic efficiency and model optimization emerged as a direct response to export restrictions on China. These constraints forced innovations that reduced hardware requirements and the number of GPU hours to train, challenging traditional scaling laws—a significant factor in valuations for AI companies, like Nvidia, that supply the hardware for AI systems. DeepSeek is believed to have pre-trained models on a 2,048 NVDA H800 cluster and took 2.79 million GPU hours1. In comparison, Meta trained Llama 3.1 405B with over 16,000 H100 GPUs and 30.84 million GPU hours3.
Scaling laws in AI suggest that a model’s performance improves predictably with increases in model size, dataset size, or both, as long as sufficient data and compute are available. A 2020 study demonstrated this trend across models ranging from 768 parameters to 1.5 billion parameters and datasets from 22 million to 23 billion tokens. The 2022 Chinchilla paper refined this understanding, showing that optimal scaling requires a 2x increase in both model and dataset size for every 4x increase in compute.
DeepSeek’s approach to AI development is not revolutionary in creating entirely new architectures but rather excels in optimizing existing methodologies with a series of incremental yet impactful improvements. As a smart-follower company, DeepSeek integrates the latest research and techniques, adapting them to enhance efficiency and performance. Their models remain transformer-based but focus on refining inefficient areas, particularly the attention block, a known bottleneck in large language models. One of their key optimizations is multi-head latent detection, which enhances training efficiency by refining how attention mechanisms distribute computational resources4. Additionally, DeepSeek effectively implements a Mixture of Experts (MoE) architecture, allowing only the most relevant portions of the model to activate per query, significantly improving inference efficiency while maintaining high performance1.
This ability to rapidly integrate cutting-edge advancements and optimize them for computational efficiency has positioned DeepSeek as a formidable contender in the AI landscape, narrowing the gap between the U.S. and China. However, it is important to note that DeepSeek is a distilled model. Distilled models are streamlined versions of larger foundation models, designed to be more efficient while retaining much of the original model’s knowledge. By leveraging this process, DeepSeek achieves impressive performance at a fraction of the cost, though it still operates as a refined derivative rather than a ground-up foundation model.
DeepSeek’s advancements are a catalyst, not signaling a disruption in AI infrastructure spending but rather a shift in how investments are allocated. Instead of curbing expenditures, DeepSeek’s efficiency-driven approach is reshaping the industry’s priorities, compelling major players to optimize resource utilization while maintaining substantial capital expenditures to meet increasing demand levels5.
Competition – AI Race Heating Up
The AI landscape is rapidly evolving, with breakthroughs coming from both established Western firms and emerging players in China. DeepSeek’s progress is seen as a signal that Chinese AI research is advancing faster than previously expected, potentially narrowing the gap with Western efforts, after China was caught off guard with the release of the initial release of ChatGPT1. Also turning the heat up, DeepSeek’s commitment to open-source AI and model commoditization is accelerating accessibility and adoption while simultaneously introducing competitive risks that challenge the established business models of major AI players. By freely sharing research and model architectures, DeepSeek is democratizing AI development, enabling more widespread innovation but also intensifying market competition. As a result, AI spending may increasingly shift toward distilled models that require fewer compute resources, optimizing efficiency without sacrificing capability. However, foundational and frontier models from industry leaders like OpenAI, Google, Anthropic, and Meta (LLaMA) will continue to drive demand for high-performance computing, as these companies push the boundaries of AI breakthroughs.
AI Infrastructure Expansion: Unwavering but Evolving
Despite DeepSeek’s claims of cost efficiency, major US hyperscalers are unlikely to alter their CapEx plans in the near term (Exhibit 1). We introduce Jevon’s paradox – Technological advancements that increase efficiency in resource use can lead to an increase in the overall consumption of that resource. Instead of decreasing resource consumption, greater efficiency can make the resource more affordable or accessible, thus driving up demand and ultimately resulting in more of the resource being used6. While training has been the focus, inference or making predictions with the model will become a bigger market over time as models mature and AI adoption increases.
In the past, running a ChatGPT query was multiple times more expensive than a Google search7. However, advancements in hardware and software have significantly reduced these costs. With DeepSeek’s innovations further lowering inference expenses, the return on investment for AI applications has become even more attractive.
As AI transitions from infrastructure to applications, investment trends will shift. Hyperscalers and startups have driven AI spending, anticipating future demand. However, with improved ROI, enterprises will ramp up their AI spending as real-world use cases expand.
We expect further disruptions, especially as reasoning models like ChatGPT O3 and Anthropic’s Claude 3.5 Sonnet require higher levels of compute. Given power and infrastucture constraints, this will drive the need for continued hardware and software optimizations, similar to DeepSeek’s innovations. As the industry digests these developments, spending may fluctuate. However, the long-term trajectory remains clear—the total addressable market is expected to continue to grow.
Exhibit 1: Capex estimates for 2025 were reaffirmed by Amazon, Microsoft, Google, and Meta
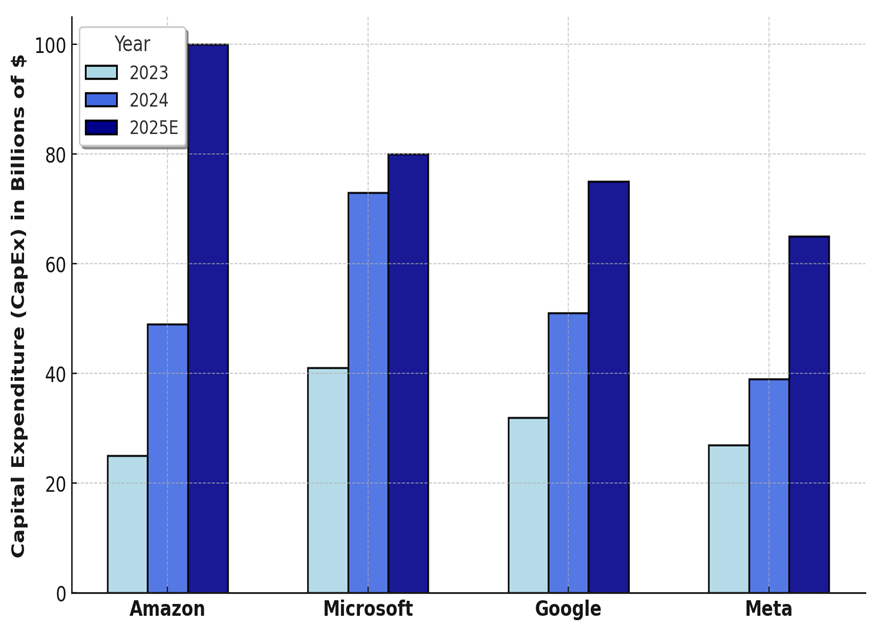
Future Trends and Research Directions
The potential for larger compute clusters in the coming years and increased processing power could unlock new AI breakthroughs. Future clusters may integrate advanced power management and networking technologies, eventually operating as a unified supercomputer-like system. Beyond hardware, software and algorithmic innovations will play a crucial role in maximizing efficiency. Advances in training algorithms, optimized data center networking, and cooling methods could further reduce costs and enhance performance. Additionally, the synergy between hardware improvements and AI training techniques is expected to drive the next wave of AI advancements.
As AI evolves, the focus is shifting from infrastructure to applications, with AI agents, enterprise use cases, and consumer adoption shaping investment returns beyond 2025. Lower inference costs could accelerate AI democratization, expanding the ecosystem of AI-driven applications and lowering barriers to software development. Edge computing is also emerging as a long-term growth driver, as more advanced edge devices may require significantly higher compute and memory to support AI workloads.
A key research question that the industry will continue to face – is how declining AI training and inference costs will influence industry-wide AI spending. Thanks to DeepSeek, this question will continue to challenge our investment thesis regarding rising AI spending.
Conclusion: Market Outlook & Investment Implications
DeepSeek’s announcement has introduced a new layer of uncertainty across the AI sector, potentially capping valuation multiples until hyperscalers offer guidance beyond 2025. Investors and industry players are closely watching how hyperscalers respond, as their strategies will shape near-term expectations for AI infrastructure investments and the AI trade.
The picks and shovels participating in the AI infrastructure buildout may face short-term headwinds if hyperscalers pause to reassess their CapEx plans. A slowdown in AI infrastructure spending could temporarily impact chipmakers and hardware providers. However, in the long run, efficiency gains from model optimizations and cost reductions could lead to even greater AI adoption, ultimately driving higher demand for AI hardware.
Meanwhile, the application and platform layers stand to benefit from increased model competition and declining compute costs. Lower barriers to entry may accelerate AI-driven innovation, creating new opportunities for software and enterprise AI solutions. Established software players are particularly well-positioned to capitalize on these trends, as reduced compute costs improve the scalability and accessibility of their AI-powered offerings.
While near-term uncertainty may lead to market volatility, the broader trajectory of AI adoption remains strong. As the industry absorbs recent developments, a clearer picture will emerge regarding AI’s long-term infrastructure needs and the resulting impact on investment trends.
Appendix A – AI Cycle
Mitchell (2019) provides an insightful explanation8:
“The two-part cycle goes like this. Phase 1: New ideas create a lot of optimism in the research community. Results of imminent AI breakthroughs are promised, and often hyped in the news media. Money pours in from government funders and venture capitalists for both academic research and commercial start-ups. Phase 2: The promised breakthroughs don’t occur, or are much less impressive than promised. Government funding and venture capital dry up. Start-up companies fold, and AI research slows. This pattern became familiar to the AI community: “AI spring,” followed by overpromising and media hype, followed by “AI winter.” This has happened, to various degrees, in cycles of five to ten years.” (p. 36)
Appendix B – Distilled Models
Distilled models are smaller, more efficient AI models created by transferring knowledge from a larger, more complex model, often called the “foundation or frontier model.” This process, known as knowledge distillation, allows for creating models that can perform well with less computational power and resources1.
- How Distillation Works:
- A large, powerful foundation model is trained on a massive dataset.
- Then, a smaller model is trained to mimic the behavior and outputs of the larger model.
- This involves transferring the knowledge and reasoning capabilities of the larger model to the smaller one.
- Techniques such as multi-layer attention (MLA) and Mixture of Experts (MoE) may be used in the distilled model for greater efficiency.
- Purpose of Distillation:
- Efficiency: Distilled models are designed to be more computationally efficient than their larger counterparts, requiring less processing power and memory. This makes them suitable for deployment on edge devices with limited resources, like phones, cars, and other devices.
- Cost Reduction: Using smaller models can significantly reduce the costs associated with training and inference (using the model to make predictions).
- Accessibility: Distillation can make AI more accessible, enabling smaller companies and individuals to use powerful AI models without requiring the massive infrastructure to train the original models.
- Improved Inference: Some distilled models are trained to think at length in response to prompts, using more computation to generate deeper answers in the inference phase. This approach, known as test-time compute, can improve the performance of distilled models. A large, powerful foundation model is trained on a massive dataset.
1 DeepSeek (n.d.). DeepSeek-V3 Technical Report. Github. https://github.com/deepseek-ai/DeepSeek-V3/blob/main/DeepSeek_V3.pdf
2 Artificial Intelligence Index Report 2024 – Stanford University. (n.d.). https://aiindex.stanford.edu/wp-content/uploads/2024/05/HAI_AI-Index-Report-2024.pdf
3 Introducing Llama 3.1: Our most capable models to date. AI at Meta. (n.d.). https://ai.meta.com/blog/meta-llama-3-1/
4 Li, S. (2025, February 10). Deepseek-V3 explained 1: Multi-head latent attention. Medium. https://medium.com/towards-data-science/deepseek-v3-explained-1-multi-head-latent-attention-ed6bee2a67c4
5 Amazon (2025, February 6). Earnings Call. Retrieved from Amazon Investor Relations website.
6 Alcott, & Hotelling, H. (2005, May 23). Jevons’ paradox. Ecological Economics. https://www.sciencedirect.com/science/article/abs/pii/S0921800905001084?via%3Dihub
7 Ars Technica. (2023, February 22). CHATGPT-style search represents a 10x cost increase for Google, Microsoft. Ars Technica. https://arstechnica.com/gadgets/2023/02/chatgpt-style-search-represents-a-10x-cost-increase-for-google-microsoft/
8 Mitchell, M. (2019). Artificial intelligence: A guide for thinking humans. Farrar, Straus and Giroux.
Disclaimer: Asset Allocation & Management Company, LLC (AAM) is an investment adviser registered with the Securities and Exchange Commission, specializing in fixed-income asset management services for insurance companies. Registration does not imply a certain level of skill or training. This information was developed using publicly available information, internally developed data and outside sources believed to be reliable. While all reasonable care has been taken to ensure that the facts stated and the opinions given are accurate, complete and reasonable, liability is expressly disclaimed by AAM and any affiliates (collectively known as “AAM”), and their representative officers and employees. This report has been prepared for informational purposes only and does not purport to represent a complete analysis of any security, company or industry discussed. Any opinions and/or recommendations expressed are subject to change without notice and should be considered only as part of a diversified portfolio. Any opinions and statements contained herein of financial market trends based on market conditions constitute our judgment. This material may contain projections or other forward-looking statements regarding future events, targets or expectations, and is only current as of the date indicated. There is no assurance that such events or targets will be achieved, and may be significantly different than that discussed here. The information presented, including any statements concerning financial market trends, is based on current market conditions, which will fluctuate and may be superseded by subsequent market events or for other reasons. Although the assumptions underlying the forward-looking statements that may be contained herein are believed to be reasonable they can be affected by inaccurate assumptions or by known or unknown risks and uncertainties. AAM assumes no duty to provide updates to any analysis contained herein. A complete list of investment recommendations made during the past year is available upon request. Past performance is not an indication of future returns. This information is distributed to recipients including AAM, any of which may have acted on the basis of the information, or may have an ownership interest in securities to which the information relates. It may also be distributed to clients of AAM, as well as to other recipients with whom no such client relationship exists. Providing this information does not, in and of itself, constitute a recommendation by AAM, nor does it imply that the purchase or sale of any security is suitable for the recipient. Investing in the bond market is subject to certain risks including market, interest-rate, issuer, credit, inflation, liquidity, valuation, volatility, prepayment and extension. No part of this material may be reproduced in any form, or referred to in any other publication, without express written permission.